
Finding short DNA motifs using permuted Markov models. In this review we discuss the general role of P-value estimation in sequence analysis, and give a description of. A rapid method for determining sequences in DNA by primed synthesis with DNA polymerase. The statistical significance of an alignment score is frequently assessed by its P-value, which is the probability that this score or a higher one can occur simply by chance, given the probabilistic models for the sequences. Complexity of strings in the class of Markov sources. A generalized hidden Markov model for the recognition of human genes in DNA. Two methods for improving performance of an HMM and their application for gene finding. Phat-a gene finding program for Plasmodium falciparum.
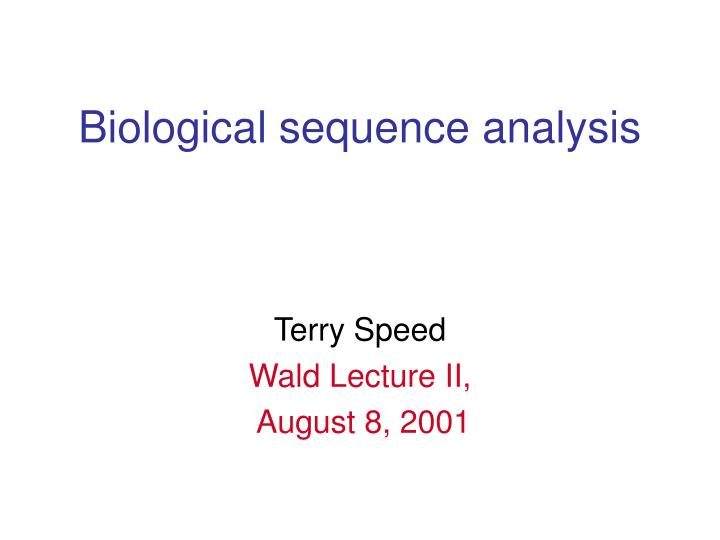
Prediction of complete gene structures in human genomic DNA. Model selection for variable length Markov chains and tuning the context algorithm.
#Biological sequence analysis software
The software implementing the gene finder was also the first HMM gene finder made available as open-source software, something of value given the rate at which new organisms were then being sequenced. This paper was also the first instance where the probabilistic formulation of the HMM gene finder was used to derive posterior probabilities of bases being part of the gene previous attempts focused exclusively on the use of the Viterbi algorithm to predict gene structures. Its novel contributions were various observations about computational shortcuts that can be made, at no cost to accuracy, taking advantage of some of the structure of the problem of applying HMMs to gene finding. The model presented was not the first of its kind similar Hidden Markov Models (HMMs) had been published before. The first, Cawley et al. , addresses the problem of analyzing stretches of DNA to search for the collections of sub-sequences that correspond to gene transcripts. The papers presented in this chapter cover two important areas in the interpretation of DNA sequences. This process is experimental and the keywords may be updated as the learning algorithm improves. These keywords were added by machine and not by the authors. I eagerly dove in to a collaboration that Terry had put in place with the Human and Drosophila Genome Projects at Lawrence Berkeley National Laboratories and spent the next few years having a great time working on interesting and practical statistical problems that arose in the context of the ongoing genome sequencing efforts. The field between computational science and biology is varyingly described as computational biology or bioinformatics. Not having thought about biology since high school, I was very impressed by the large impact statistical approaches were making in a field I had naively considered as one that had little to do with quantitative analysis. Berkeley Statistics department in 1995, I had the good fortune to meet Terry and learn about some of his work in the area of the application of statistics to genetics and molecular biology. Shortly after the start of my graduate studies at the U.C.
